According to the World Health Organization, an estimated 1.25 million pedestrian fatalities are registered annually worldwide (World Health Organization, Global Status Report on road safety). Road traffic accidents are thought to cost most countries about 3% of their gross domestic product. Without sustained action, it is projected that road traffic crashes will become the seventh leading cause of death by 2030.
When planning road safety policies for the protection of pedestrians, one cannot disregard the forthcoming deployment of autonomous vehicles. The autonomous vehicles are expected to resolve some of the major reasons behind pedestrian fatalities, such as speeding and distracted driving. Pedestrian behavior, however, is complicated, at times stochastic and chaotic especially in highly dense urban centers. Hence, developing algorithms capable of predicting pedestrian intention is among the main challenges facing car manufacturers today.
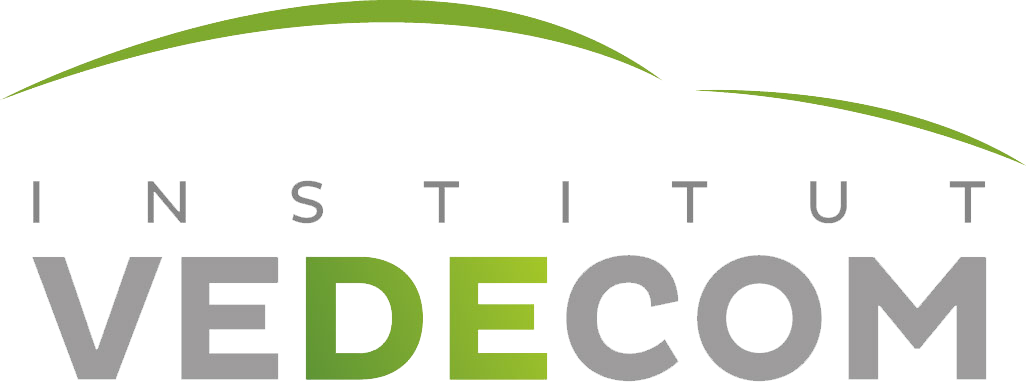
VEDECOM decided to employ an interdisciplinary research team to tackle the challenge. The team is composed of bio-mechanists who are specialized in human locomotion, alongside computer scientists who aim at developing neural networks to predict future trajectories of pedestrians based on prior knowledge about their posture and movements. While human locomotion has been extensively studied in gait laboratories, proper examination of pedestrian behavior requires acquiring motion capture data outdoors, in ecological environments. For this purpose, we carry out research with an automated vehicle prototype of VEDECOM on a test track. We use the Xsens system to capture pedestrian kinematics. We can easily synchronize the MVN Awinda with other sensors onboard the automated vehicle, such as camera and Lidar, as well as GPS data.
The results obtained from the kinematic measurements of pedestrians crossing the street seem to highlight distinct motor control programs. The fusion of the acquired sensory information helps to estimate the position and velocity of the pedestrian relative to the car. Currently, we are in the post-processing phase. The goal is to teach the vehicle embedded systems to identify crossroad motor patterns that were captured using the MVN Awinda.