Authors: João Quintas1,2, Kamrad Khoshhal1, Hadi Aliakbarpour1, Jorge Dias1
1 - Institute of Systems and Robotics, University of Coimbra
2 - Institute Pedro Nunes
Background
Human behavior modeling is one of the biggest challenges in artificial intelligence science. There are many applications related to this subject for example; surveillance systems (e.g. airport, bank, train station, etc.), virtual reality (e.g. interactive virtual worlds, virtual studios teleconferencing, etc.), motion analysis (e.g. choreography of dance, clinical studies of orthopedic patients, etc.) and Human-Robot Interaction (HRI).
We present the example for fall detection, to better understand the importance of the studies in the area of human behavior analysis. Falls are among the top causes of unintentional injury and death in the elderly population. Several studies highlight the social impact of this problem, which reach global scale. Falls in older people are estimated to affect 30% of those over 65 on an annual basis. Although most falls procure no injury, between 5-10% of elderly people who fall each year sustain serious injury, such as fracture, head trauma, or serious laceration. Of the estimated 1% of the elderly who fall and sustain a hip fracture, 20-30% die within one year of the fracture. As many as two thirds of elderly with hip fracture never regain their pre-fracture activity status and one-third require nursing home placement.
Given these facts, human behavior analysis can contribute with a strong point both on the prevention and detection of this type of hazardous situation. Systems monitoring the elderly living space could analyze potential risks of falls occurring and identify potential causes of falling and consequently correct adaptations on the living space. In terms of fall detection, it would be advantageous, for those situations where full monitoring is not possible, to have systems with the capabilities for alarming carers about abnormal situations.
Methods
One of the common approaches to obtain the input data for applying human behavior analysis techniques is using motion trackers which are more precise than the other trackers.
We use MVN Analyze® to collect the data related with position and acceleration of some body parts, with the intent of using these data as ground truth for that obtained by a multi-sensor multi-modal framework.
The applied approaches aim to extend the application of Laban Movement Analysis, commonly used as notation for human movement description in dance, to the field of Human Behavior Analysis.
Features are extracted and analyzed in the frequency domain using Digital Signal Processing (DSP) techniques (e.g Fast Fourier Transform (FFT)).
After features are extracted they are fed to a Bayesian Network that performs the analysis and classification of LMA parameters resulting in the identification of the action/ behavior being performed.
Experiment
We attempted to apply our method to understand sequence of human actions through a long signal data which can include different actions. For this reason, we need to define a window-frame on the signal, in order to better discriminate the actions in case they are executed in a very short time apart.
We used a dataset comprised of more than 100 different collected samples (human actions) and analyzed each body part.
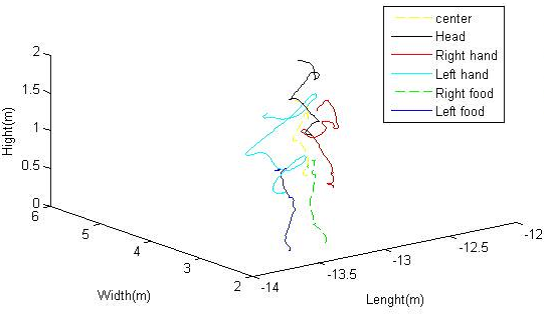
Figure 1 Position sequences for the 6 body parts for running action in 3D view
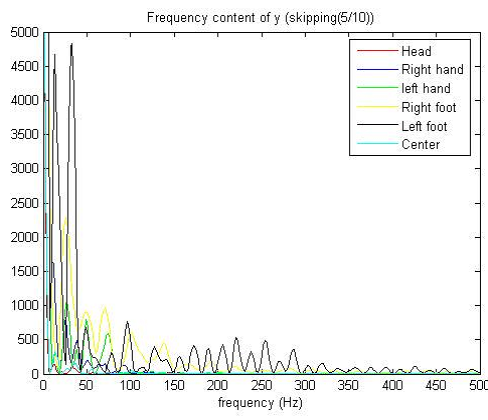
Figure 2 3D acceleration signal of the 6 body parts for skipping action
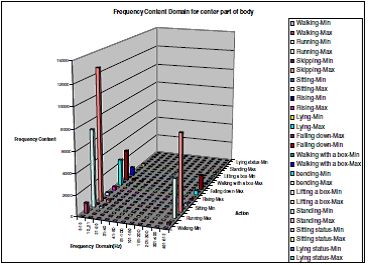
Figure 3 Histogram for the center part of the body, showing the definition of some frequency subdomains on the power spectrum signals
Conclusion and future work
A two-step probabilistic approach was presented that aims to understand human actions and behaviors based on the LMA descriptor and using features which are extracted from 3D acceleration data from different parts of the body. The first level is to obtain the LMA parameters and the second one is for action understanding part.
Acknowledgments
We would like to thank Luis Santos and Paulo Freitas for their contribution. This work has been supported by the European Union within the FP7 Project PROMETHEUS.
References
[1] K. Khoshhal, H. Aliakbarpour, J. Quintas, P. Drews, J. Dias - Probabilistic LMA-based Classification of Human Behaviour Understanding Using Power Spectrum Technique - Fusion 2010 - 13th International Conference on Information Fusion - EICC Edinburgh, UK, July 26-29 2010.
[2] K. Khoshhal, H. Aliakbarpour, J. Quintas, K. Mekhnacha, J. Ros and J. Dias - "LMA-based Human Behaviour Analysis Using HMM" - In DoCEIS’11 , 2nd Doctoral Conference on Computing, Electrical and Industrial Systems, 21-23 February 2011, Costa da Caparica, Lisbon, Portugal.
[3] K. Khoshhal, H. Aliakbarpour, J. Quintas, M. Hofmann and J. Dias - "Probabilistic LMA-based Human Motion Analysis by Conjugating Frequency and Spatial based Features" - in Wiamis 2011, 12th international Workshop on Image Analysis for Multimedia Interactive Services (WIAMIS), 13-15 April 2011.
Are you interested our solutions? Please click on the button below to contact us.
