V.L. Fuschillo1,2, F. Bagalà1*, L. Chiari1,2, A. Cappello1,2
1Department of Electronic, Computer Science and Systems, University of Bologna
2Health Sciences and Technologies Interdepartmental Center for Industrial Research (HST-ICIR), University of Bologna
* corresponding author: fabio.bagala@unibo.it
Introduction
The trajectories of the body Center of Pressure (CoP) and the body Center of Mass (CoM) are commonly investigated in studies of human posture and balance control [1,2,3,4] and in many functional tests [5,6,7]. While the CoP can be measured by means of a force plate (FP), the whole body CoM location is not directly observed and must be estimated. This application aims to present a novel, functional, model-based approach to predict Center of Pressure and Center of Mass during sit-to-stand and postural oscillation tasks using accelerometry only. These motor tasks are routinely used in clinics for multiple purposes (e.g., evaluation of postural control, risk of fall, lower-extremity strength, and impairment after stroke). The application here presented allows the kinematics and dynamics to be predicted using a simple, accurate, and portable setup, speeding up the experimental sessions and reducing the computational and economic costs, especially when several subjects are involved.
Methods
Three young healthy subjects were asked to perform two different motor tasks: five trials of ten repeated sit-to-stand on a chair and five trials of voluntary postural oscillations, using a pure ankle strategy as much as possible, standing on a FP (Bertec 4060-08). In both tasks, three MTx (Xsens Technologies, Netherlands) were placed at measured heights with respect to the ankle, knee, and hip joint, respectively. Each sensor was mounted on the skin, in a central position on the lateral side of the thigh and the shank, and on the posterior side of the head-arms-trunk, to minimize skin artifact effects and model errors. For the present study, only the accelerometer output related to the axis orthogonal to the body segment is acquired. FP and accelerometer signals were acquired at a 100Hz sampling rate and low pass filtered (2nd order zero-phase Butterworth filter) at a cut-off frequency of 3Hz.
First, the anthropometric parameters of the subjects were estimated through the method presented in [8] using a three-link biomechanical model, a FP and accelerometry. The sway angles were estimated using a bidirectional filtering of the accelerometer outputs (cut-off frequency depending on the position of the sensor) as presented in [9]. The method showed a good accuracy (less than 1°) with respect to a stereo-photogrammetric system.
Finally, the Ground Reaction Force, the displacement of the CoP in the AP direction, the displacement of the CoM in the AP and vertical directions were predicted using only the three outputs of the sensors and the estimated anthropometric parameters, during the sit-to-stand and oscillatory trials. Dynamics variables were compared with the FP outputs.
Results
A qualitative evaluation of the FP outputs and residuals between measured signals and signals predicted by accelerometry is reported in Fig. 1 for one rSTS trial and in Fig. 2 for one oscillatory trial of one of the subjects. RMSE of CoP, averaged over the three subjects, is 21.1mm with a mean peak-to-peak range of 401.7mm for the sit-to-stand and 5.5mm with a mean peak-to-peak of 21.5mm for the oscillatory trials.
Discussion
First, it is important to note the effectiveness of the estimation procedure, which allows the evaluation of the subject-specific anthropometric parameters by means of a simple motor task (repeated sit-to-stand) involving only a few accelerometers and a FP. Moreover, the estimated parameters can easily be applied to different motor tasks sharing the same biomechanical model. Second, after this preliminary estimation, the suggested method provides the prediction of Ground Reaction Force, CoP and CoM during sit-to-stand, postural oscillation, squatting, etc. using only accelerometry, which compares favorably to commercial stereo-photogrammetric movement analysis systems in terms of cost, size, weight, convenience and portability. As a result, data collection is no longer confined to a laboratory environment.
Reference
[1] Horak FB (1997) Clinical assessment of balance disorders. Gait Posture 6(1): 76-84
[2] Iqbal K (2011) Mechanisms and models of postural stability and control. Proceedings of the 33rd Annual International Conference of IEEE Engineering in Medicine & Biology Society, Boston, pp 7837-7840
[3] Prieto TE, Myklebust JB, Hoffman RG, Lovett EG, Myklebust BM (1996) Measures of postural steadiness: differences between healthy young and elderly adults. IEEE T Bio-Med Eng 43: 956-966
[4] Winter DA (1995) Human balance and posture control during standing and walking. Gait Posture 3: 193-214
[5] Fujimoto M, Chou LS (2012) Dynamic balance control during sit-to-stand movement: an examination with the center of mass acceleration. J Biomech 45: 543-548
[6] Pai YC, Naughton BJ, Chang RW, Rogers MW (1994) Control of body center of mass momentum during sit-to-stand among young and elderly adults. Gait Posture 2: 109-116
[7] Papa E, Cappozzo A (1999) A telescopic inverted-pendulum model of the musculo-skeletal system and its use for the analysis of the sit-to-stand motor task. J Biomech 32: 1205-1212
[8] Fuschillo VL, Bagalà F, Chiari L, Cappello A (2012) Accelerometry-based prediction of movement dynamics for balance monitoring. Med Biol Eng Comput, DOI 10.1007/s11517-012-0940-6
[9] Bagalà F, Fuschillo VL, Chiari L, Cappello A (2012) Calibrated 2D angular kinematics by single-axis accelerometers: from inverted pendulum to N-link chain. IEEE Sensors Journal 12(3): 479-486
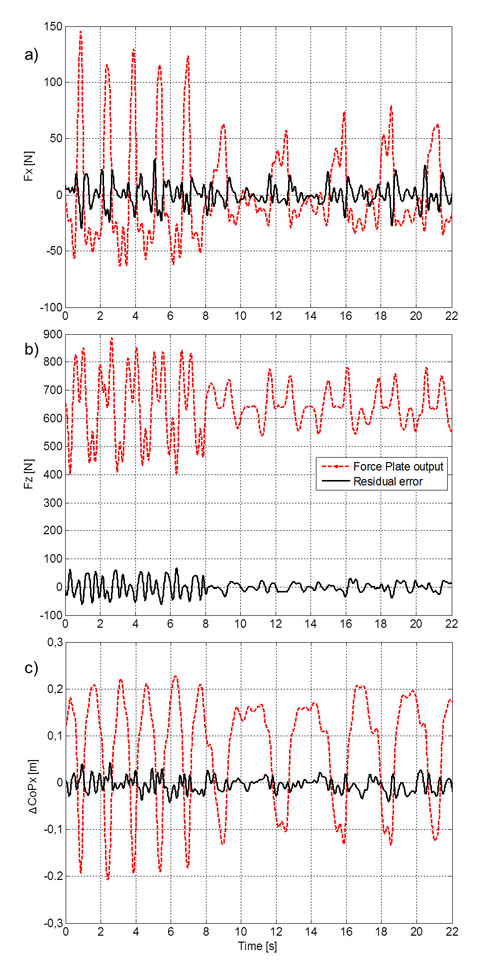
Fig. 1 a) FX, b) FZ, c) ΔCoPX patterns (red lines) and residual prediction errors (black lines) for a rSTS trial
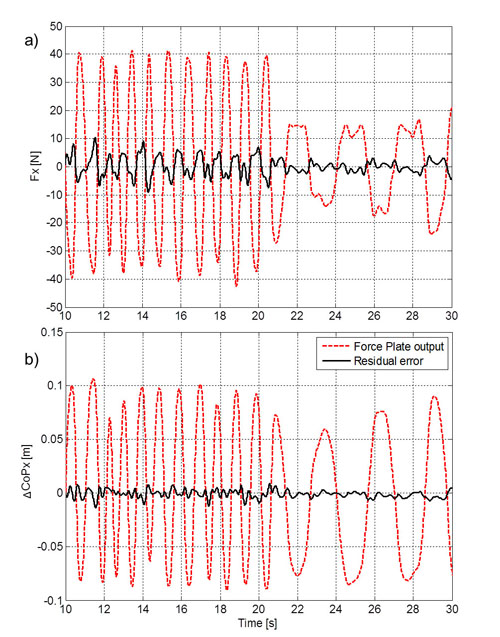
Fig. 2 a) FX, b) ΔCoPX patterns (red lines) and residual prediction errors (black lines) for an oscillatory trial
Are you interested our solutions? Please click on the button below to contact us.
